Wondering how incrementality experiments differ from A/B tests, and when to use one over the other? You’re in the right place.
A/B testing is a go-to tactic for many marketers; in fact, over 70% believe it’s essential for improving conversion rates.
But it doesn’t always tell the full story. Just because version B beats version A doesn’t mean your campaign actually drove new growth.
That’s where incrementality testing steps in; it helps you understand whether your marketing activity truly caused a lift in performance, or if the results would’ve happened anyway.
In this blog, we’ll cover:
- What A/B testing and incrementality testing really mean (with examples)
- How their goals, designs, and outcomes differ
- When to use each method based on what you're trying to prove
- Common types of incrementality testing
- Key challenges and what to watch for in both approaches
P.S. Not sure if your marketing is driving real growth or just making things look better on the surface? inBeat Agency helps you launch data-backed campaigns using creators and paid media that deliver true, measurable impact, not just clicks. Book a free strategy call now and let’s scale smarter.
TL;DR:
A/B Testing compares different versions of a single element (e.g. ad copy, CTA) to improve performance metrics like click-through or conversion rates.
Incrementality Testing measures the true causal impact of a campaign by comparing a test group (exposed to ads) against a control group (not exposed).
A/B is great for tactical optimizations; incrementality is better for strategic decisions like budget allocation and channel effectiveness.
A/B tests are faster, simpler to run, and need smaller audiences; incrementality tests require more planning, longer timeframes, and larger samples.
Common types of incrementality tests include geo-testing, known audience testing, platform-led conversion lift studies, and observational experiments.
Use A/B testing to refine elements like landing pages, visuals, and messaging.
Use incrementality testing to prove long-term value, measure upper-funnel impact, or decide where to scale spend.
Each method has challenges: A/B can mislead if it ignores external factors, while incrementality testing needs careful design to avoid bias.For best results, use both approaches in tandem to build smarter, data-backed marketing strategies.
What Is A/B Testing?
A/B testing, also known as split testing, is a straightforward method where you compare two versions of something, like an ad, webpage, or app, to see which one performs better.
You randomly split your audience into two groups: one sees version A, the other sees version B. Then you measure the outcome using performance metrics like conversion rate, click-through rate, or bounce rates.
This kind of testing focuses on direct comparisons between variations to improve a single marketing element. It’s especially useful when you're optimizing user experience or fine-tuning details like hooks, subject lines, or call-to-action buttons.
But while A/B testing shows which version performs better, it doesn’t tell you whether the entire marketing activity had any real impact; that’s where incrementality testing comes in.
What Are Incrementality Experiments?
Incrementality experiments are randomized, controlled tests used in marketing to isolate the true effect of a campaign. They’re built to help you figure out what would have happened without your marketing activity.
Instead of just comparing two versions of an ad or webpage, incrementality testing looks at the true impact of your marketing activity by measuring the additional conversions driven by the campaign. That’s the incremental lift.
To do this, you create a control group that sees no ads at all and compare it to a test group that does. If the test group performs better, that difference is your incremental impact. This method helps marketers understand the causal impact of an entire advertising campaign, not just tweaks to a single element. It’s especially useful in a privacy-first era, where traditional tracking can miss the full story.
“Incrementality testing has become the industry’s gold standard for understanding advertising’s true impact in a privacy-first way.” JD Ohlinger, Nik Nedyalkov - Think with Google
Read Next: Incrementality Testing: Calculations, Examples, and More
How Are Incrementality Experiments Different From A/B Experiments
A/B experiments compare two versions of a single marketing element, e.g., different headlines in an ad, to see which performs better. Incrementality experiments measure whether a campaign drove results that wouldn’t have happened otherwise. A/B testing optimizes elements, while incrementality testing reveals the true impact of marketing efforts.

Objective
- A/B testing: Focuses on identifying which version of a specific marketing element performs better. This could be anything from a headline or image to an action button or subject line. The goal is to improve user engagement and boost performance metrics by changing a single campaign element.
For example, for our client Hurom, we ran an A/B test using the same product image but different ad copy and CTAs to see which messaging resonated more with the audience.
- Version A focused on "transformation," highlighting benefits like strengthening immunity, supporting weight loss, and energizing the body. Its CTA encouraged users to "Start your journey today."

- Version B emphasized "health empowerment," promoting immunity boost, gut health support, and effortless daily juicing. Its CTA urged users to "Take control of your health today."
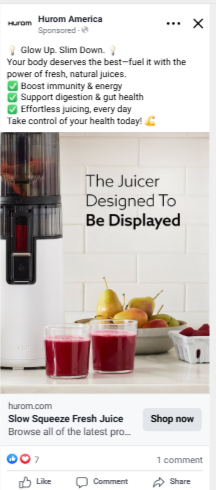
This test helped us gather insights on which emotional triggers, transformation versus empowerment, and which CTA styles drove higher engagement and conversions.
- Incrementality testing: Measures the actual contribution of a marketing activity to business outcomes. Instead of comparing versions, it reveals whether the campaign itself drove additional impact, such as more conversions, app downloads, or sales, beyond what would have happened without it.
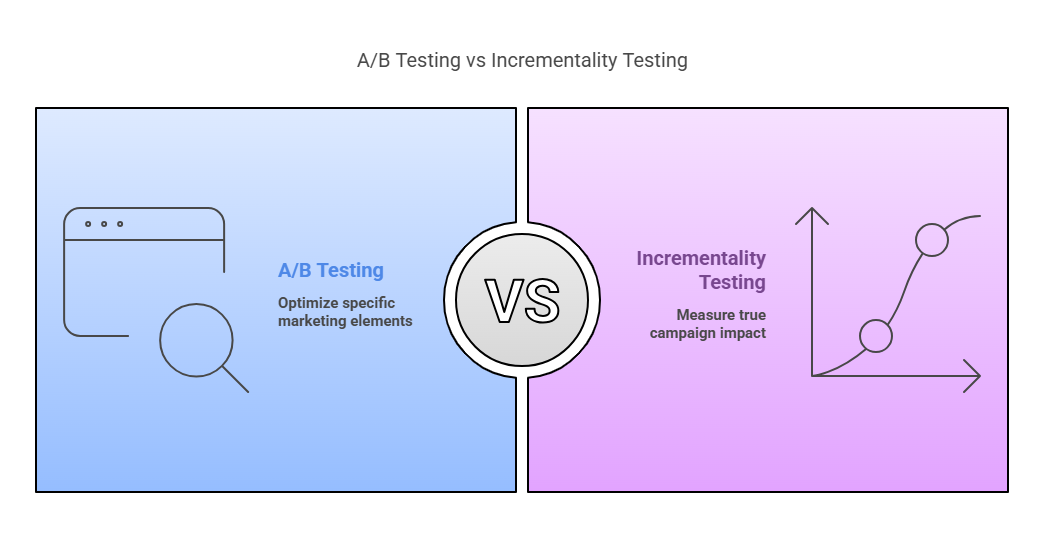
Scope
- A/B testing: Narrow in focus. Ideal for making tactical improvements based on user behavior.
- Incrementality testing: Broad in scope. It helps marketers answer bigger strategic questions about budget allocation, channel performance, and the true impact of their marketing efforts.
Methodological Approach
- A/B testing: Compares two or more variants (like version A and version B) by showing them to similar audience groups at the same time. The goal is to see which version performs better based on key metrics like click-through rate or conversion rate.
- Incrementality testing: Uses a control group that doesn’t see the campaign at all, and compares it to a test group that does. This setup isolates the incremental lift, helping you measure the true impact of your marketing activity, without interference from external factors or pre-existing behavior.
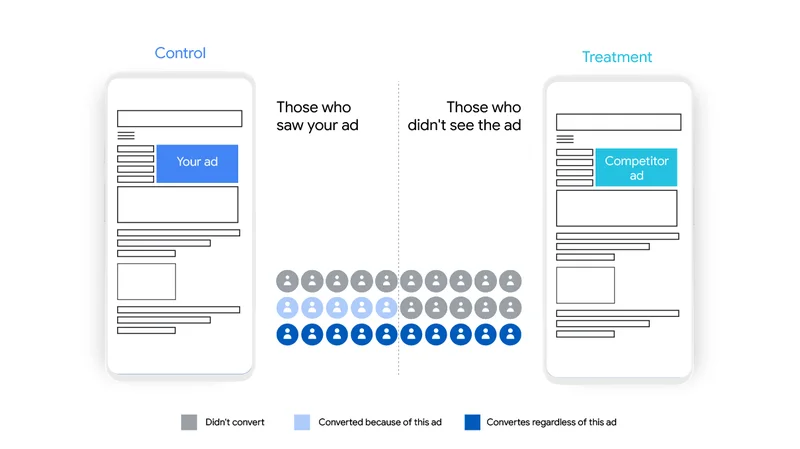
Measurement and Metrics
- A/B testing: Tracks performance-focused metrics like clicks, open rates, bounce rates, and conversion rate. These metrics help you understand how well each version of a marketing element is engaging users.
- Incrementality testing: Focuses on business-impact metrics such as net-new conversions, incremental revenue, customer acquisition, and lift in conversions. The goal is to measure outcomes that were directly caused by your marketing campaign, not just correlated with it.
Sample Size
- A/B testing: Can work with smaller samples when testing high-frequency events like click-through rate or email opens. However, for low-conversion events like purchases or app installs, you’ll need a larger audience to get reliable, statistically significant results and avoid misleading data.
- Incrementality testing: Requires larger sample sizes by default. Since you're measuring the additional impact of your marketing activity against a control group, a bigger audience is needed to ensure statistical accuracy and detect a meaningful incremental lift.
Timeframe
- A/B testing: Typically runs over a short period, anywhere from a few hours to a few days, especially when you're testing high-traffic elements. It’s designed for quick insights and fast iteration on specific parts of your marketing campaign.
- Incrementality testing: Requires a longer timeframe, usually several weeks or more. Since it measures the real impact of a marketing activity over time, it needs enough duration to account for external factors, reach statistical significance, and capture the full effect on user behavior and business metrics.
Different Types of Incrementality Testing
Now that we’ve covered how incrementality testing differs from A/B testing, let’s look at the various ways marketers actually run these experiments in the real world.
Each method has its strengths, depending on your goals, data access, and channels.
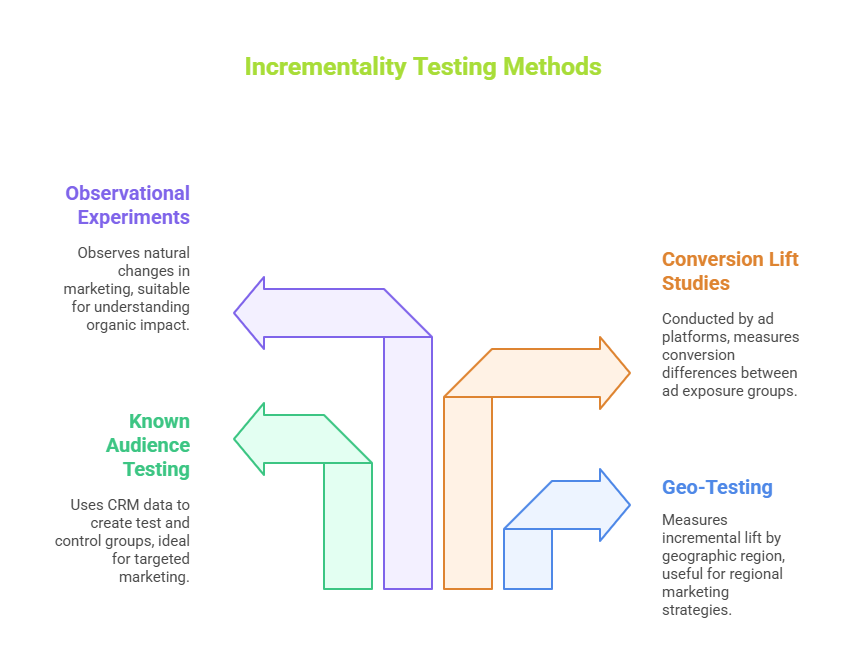
1. Geo-Testing
This approach splits your audience based on geographic regions; for example, running a campaign in one city while withholding it in another. It’s especially useful when cookie-based tracking or user-level attribution isn’t reliable.
Geo-testing helps measure incremental lift at the regional level, making it a strong option for large-scale advertising campaigns where individual tracking is limited or restricted.
2. Known Audience Testing
This method uses your CRM data or other first-party data to create test and control groups. Since you already know who your audience is, you can split them intentionally so that some see your marketing activity and others don’t.
It’s a great way to measure the causal impact of a campaign on user behavior, especially when you're running email, app, or loyalty-based campaigns. Since you're working with known users, it offers more control and precision, making it easier to tie outcomes like conversions or app downloads directly to your advertising efforts.
3. Conversion Lift Studies
These are usually run directly by digital ad platforms like Meta (Facebook Lift), TikTok or Google. The platform splits users into a test group that sees your ads and a control group that doesn’t, then measures the difference in conversions.
It’s a powerful way to understand the incremental impact of your advertising efforts without needing to manage the test manually. These studies are especially useful when you're running large-scale campaigns and want a clear view of how your ads influence consumer behavior and drive additional conversions.
4. Observational/Natural Experiments
These tests rely on real-world situations, like organic pauses in campaigns, regional rollouts, or platform outages, to observe what happens when marketing stops or changes naturally. There’s no formal setup like a control group, but by analyzing the timing and location of changes, marketers can still uncover the causal impact of their marketing activity.
While less precise than controlled tests, observational experiments are helpful when you can't run a structured test but still want to understand the real impact of your advertising efforts.
Marketing expert Michael Kaminsky shares practical ways you can use incrementality testing to determine if your brand search spend is actually driving incremental results or just eating up budget.
Understanding the different types of incrementality testing is important, but knowing when to use A/B testing vs. incrementality testing is what really helps you make data-driven decisions.
Let’s break down when each method makes the most sense.
When to Use A/B or Incrementality Testing Method
A/B testing is best for optimizing specific elements of a campaign, while incrementality testing is used to measure whether the campaign itself caused meaningful results.
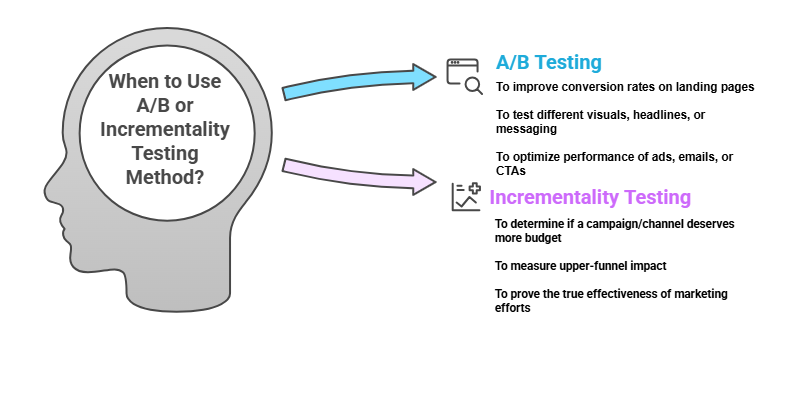
Use A/B Testing When You Want To:
- Improve conversion rates on landing pages: Test layout, copy, or design variations to see which leads to more form fills or purchases.
- Test different visuals, headlines, or messaging: Compare creative elements to see which one captures more attention or drives better engagement.
- Optimize performance of ads, emails, or CTAs: Make small, data-backed changes to boost click-through rate, open rate, or conversions on specific marketing assets.
Pro tip: inBeat.co’s free ad mockup generator lets you preview and A/B test different ad creatives across multiple platforms to see what fits best.

Use Incrementality Testing When You Need To:
- Determine if a campaign or channel deserves more budget: Find out if your marketing spend is actually driving net-new results before scaling.
- Measure upper-funnel impact (e.g. display ads, YouTube, awareness ads): Evaluate campaigns where the goal isn’t an immediate conversion, but long-term influence on brand or behavior.
- Prove long-term value and the true effectiveness of marketing efforts: Understand whether your campaigns are creating sustainable growth, acquiring new customers, or driving revenue, not just shifting existing demand.
Choosing the right method is important, but it's just as critical to understand the challenges that come with each. And that takes us to the next point.
Incrementality Testing vs A/B Testing: Challenges and Considerations
Both A/B testing and incrementality testing are valuable tools, but they come with their own trade-offs. Understanding these challenges helps you pick the right approach and set realistic expectations for what the results can (and can’t) tell you.
Incrementality Testing
- Requires more upfront planning, larger audiences, and sometimes a bigger budget to run effectively.
- Results can be influenced by external factors like seasonality, promotions, or competitor activity, which can make it harder to isolate the true impact of your campaign.
- Typically runs over a longer period and may not provide real-time insights like an A/B test can.
- Needs solid experimental design to avoid biased results or misinterpreting incremental lift.
A/B Testing
- Easier to set up and often delivers faster results, but it doesn’t account for outside variables that might influence performance.
- Can give a false sense of success. Just because version B outperforms version A doesn’t mean it’s driving net new growth, it could just be shifting results within the same audience.
- Works best for micro-optimizations, but not for answering bigger business questions like whether a marketing channel deserves more investment.
Run Smarter Campaigns With inBeat Agency From A/B to Incrementality Testing
Whether you’re optimizing a headline or evaluating the true impact of an entire campaign, choosing the right testing method is key. A/B testing helps fine-tune your assets, while incrementality testing reveals whether your marketing efforts are truly driving growth. Knowing when and how to use each approach can lead to more informed decisions and better results.
Key takeaways:
- A/B testing compares two or more versions of a specific element to improve performance.
- Incrementality testing measures the additional impact caused by a marketing campaign.
- A/B is ideal for fast, tactical optimizations like CTAs, headlines, or ad creatives.
- Incrementality is better for evaluating long-term impact and justifying budget allocation.
- A/B tests can miss external factors that affect results; incrementality testing accounts for them.
- Incrementality tests need larger audiences and longer durations for reliable insights.
- Tools and setup differ: A/B is simpler; incrementality needs more planning and scale.
- Use both testing methods strategically to build data-driven, sustainable marketing strategies.
If you're looking to build campaigns that don’t just look good in reports, but actually drive new growth, inBeat Agency can help. Our team blends creator-powered content with paid media and the right testing frameworks to deliver results you can measure and scale.
Book a free strategy call now and let’s build your next high-impact campaign!
Frequently Asked Questions (FAQs)
What is the main goal of incrementality testing?
The goal of incrementality testing is to measure whether a marketing activity caused real, additional impact, such as new conversions, revenue, or customer acquisition, that wouldn’t have happened otherwise. It helps marketers understand the true effectiveness of their campaigns.
Can A/B testing measure the overall impact of a marketing campaign?
Not really. A/B testing tells you which version of a specific element performs better (like an ad headline or landing page layout). It doesn’t account for external factors or measure whether the campaign itself drove net new results. That’s where incrementality testing comes in.
How do I decide whether to use A/B testing or incrementality testing for my campaign?
It depends on your objective. If you're optimizing a single element (like improving click-through rate), go with A/B testing. If you want to understand whether your entire campaign or channel investment is actually driving results, incrementality testing is the better choice.
What are the key differences between A/B testing and incrementality experiments?
A/B testing compares versions of one element to find the top performer. Incrementality testing compares exposed vs. unexposed audiences to determine if your marketing had any additional impact. A/B is for optimization, incrementality is for proving value.
Which types of businesses can benefit from incrementality testing?
Any business running digital marketing campaigns at scale, especially those investing across multiple channels like paid social, display, or influencer marketing. It's especially valuable for e-commerce, subscription models, mobile apps, and brands focused on growth and attribution.
What kind of data is required to run an incrementality test?
You’ll need access to performance data like conversions, revenue, or installs, along with the ability to segment audiences into test and control groups. First-party data, CRM lists, geo-targeting, or platform tools (like Meta Lift) are often used to set up the test.
Is incrementality testing effective for B2B companies?
Yes, especially when B2B brands run longer sales cycles or multi-touch campaigns. Incrementality testing can help determine whether a marketing channel or campaign is truly influencing leads, pipeline, or closed deals, rather than just taking credit for what was already in motion.